Financial modelling
Stock price climbs in anticipation of good earnings. If earnings per share (EPS) beat Wall Street expectations, then depending on Surprise %, the price changes. The black dot represents the earnings report release. Image generated using Python.
In this project, my goal is to model and improve my investment strategy. I test the flaws, strengths and limits of the strategy.
My research led me to Quantopian. It is an online platform that enables one to program trading strategies in Python and then back-test, live-test, live-trade and possibly license the same strategies. My draw to Quantopian was the availability of free-of-cost minute-by-minute stock price data and other data.
Through experience, I learned that programming the trading strategy outside the Quantopian platform can be very time-consuming. With your own code, you can be bogged down in tedious tasks such as fixing dates (line #56), plotting results (line #66), etc instead of focusing on the actual trading algorithm. I share a portion of my home-brewed program to show how tedious it can be. One can use zipline package to ease the pain.
Data acquisition
Using Python and iMacros scripts, I downloaded earnings calendars of 2,143 US companies starting from 1998 to 2020.
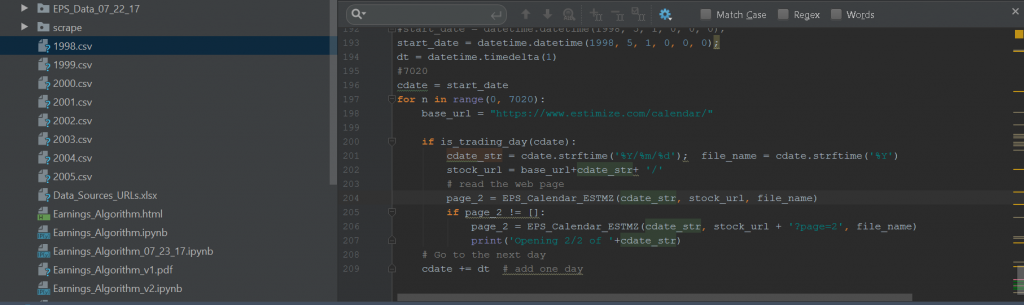
Screenshot showing one of many Python data-mining scripts I used to download earnings calendars. YES, I read and parsed over 20,000 html pages !! Thanks to Beautiful Soup.
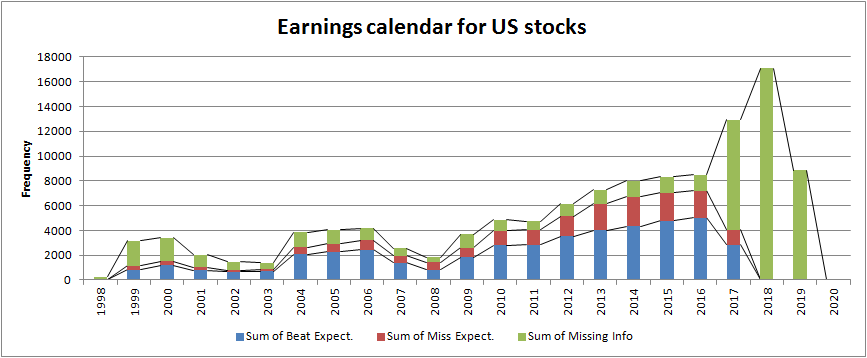
Summary of my earnings database. I still have missing information on 54,076 earnings releases (e.g. Wall St. expectations and actual EPS); a fraction of those have future release dates, i.e., Q3 and Q4 of 2017, 2018, and 2019. Of the 64,588 earnings releases with complete info, 70% beat market expectations.
Trend analysis
For a select companies, I analyzed historical stock prices around the earnings calendar. I find a few observable trends before and after earnings releases as I demonstrate in the figures below:
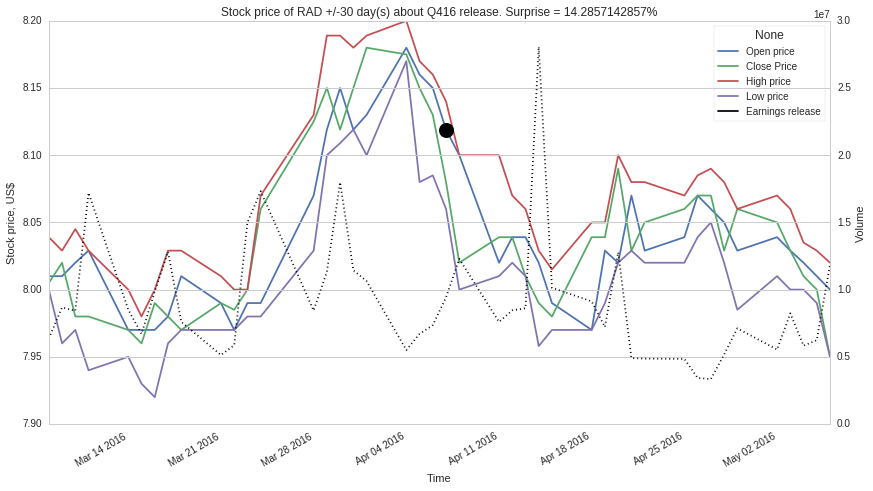
Stock price climbs in anticipation of good earnings. If earnings per share (EPS) beat Wall Street expectations, then, depending on Surprise %, the price changes.
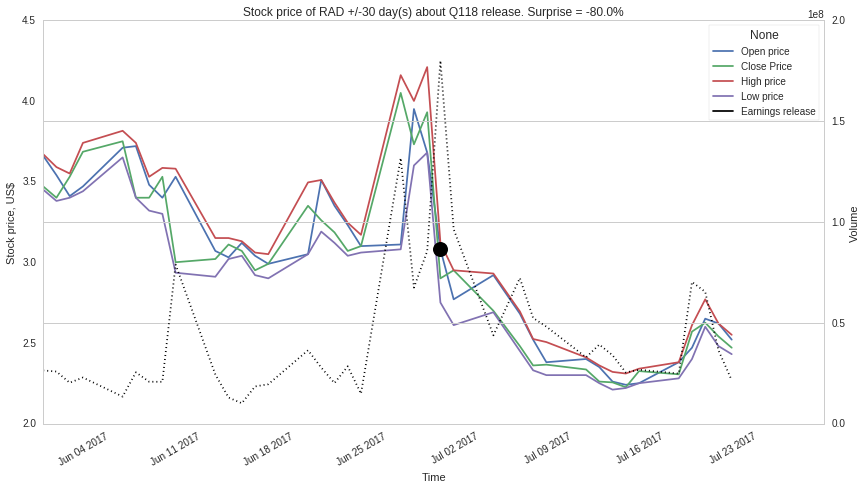
Stock price climbs in anticipation of good earnings. If earnings per share (EPS) beat Wall Street expectations, then, depending on Surprise %, the price changes.
Regression
Here, I mathematically derived 3 signals to identify optimum buy and sell times before earnings release day. Some traders widely use the Moving Average Convergence Divergence (MACD) signal.
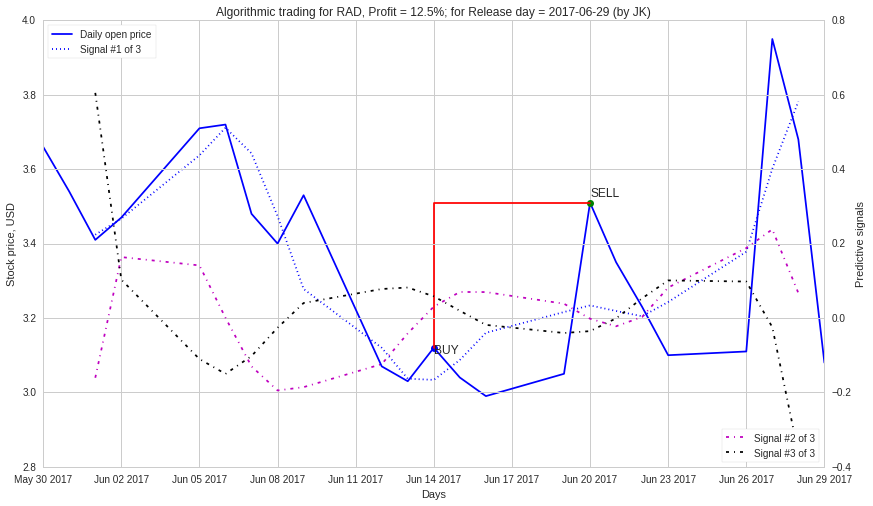
Simulated trade using my earnings-based algorithm. The buy/sell trades are executed days before a the release of quarterly earnings report. I use the signals (dotted lines) to decide.
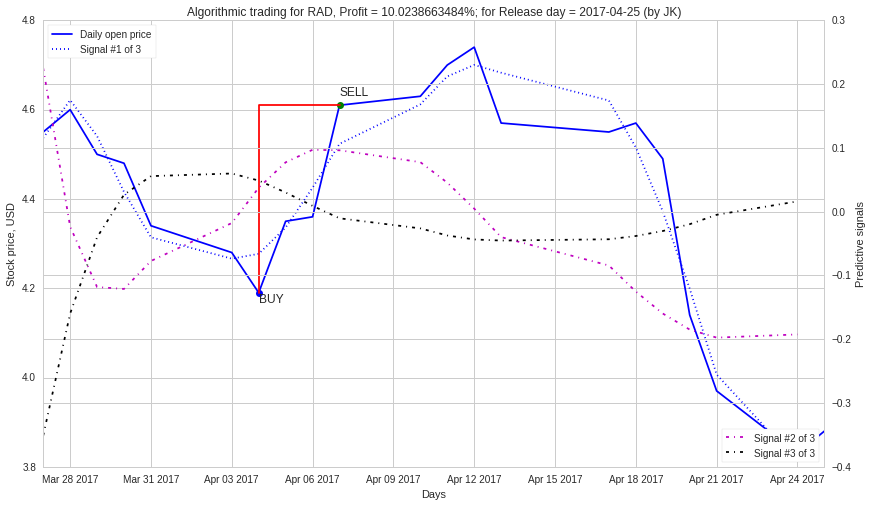
Sample trade using my earnings-based algorithm. The buy/sell trades are executed days before a the release of quarterly earnings report.
Back-testing
I am currently back-testing my earnings-based algorithm on Quantopian’s Python-based platform. A sample result is shown in the plot below. The platform executes the algorithm over a specified period, starting capital (US$ 10,000.00), type of securities, a set of stocks, commission and other brokerage fees and of course, the user-generated algorithm written in Python. Quantopian then outputs the total returns of the algorithmic trades among other variables.
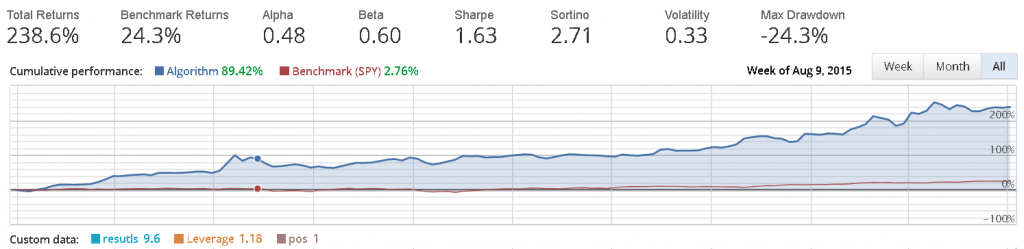
Sample back-test results I did with a test algorithm from Jan-2015 to Jul-2017. Here, I used minute stock price data available on Quantopian. I use this platform to test trading strategies.
Example code of programming for finance in Python |
1 # Author: Jonah Kadoko 2 # Date: 07-19-17 3 # Description: 4 # The goal of this project is to be able to test specific trading algorithms using Python 5 6 import matplotlib.pyplot as plt 7 import matplotlib.dates as mdates 8 import datetime 9 import numpy as np 10 import urllib 11 12 def month2num(month): 13 # this function changes the month from letters to numbers 14 month_list ={ 15 'Jan' : 1, 16 'Feb' : 2, 17 'Mar' : 3, 18 'Apr' : 4, 19 'May' : 5, 20 'Jun' : 6, 21 'Jul' : 7, 22 'Aug' : 8, 23 'Sep' : 9, 24 'Oct' : 10, 25 'Nov' : 11, 26 'Dec' : 12 27 } 28 return month_list[month] 29 30 def stock_data(ticker): 31 fig = plt.figure() 32 ax1 = plt.subplot2grid((1,1), (0,0)) 33 # ticker = 'GOOG' 34 stock_price_data = [] 35 open_p = []; vol = []; close_p = []; trade_date = [] 36 stock_price_url='https://www.google.com/finance/historical?output=csv&startdate=Jul+20+2008&enddate=Jul+19+2017&q='+\ 37 ticker 38 raw_data = urllib.request.urlopen(stock_price_url).read().decode() 39 split_raw_data = raw_data.split('\n') 40 print(split_raw_data[0]+'\n') 41 42 for line in split_raw_data: 43 split_line = line.split(',') 44 if '\ufeff' in line or len(split_line) != 6: 45 print('debug_1') 46 else: 47 # print('debug_2') 48 # print(split_line[1]) 49 # stock_price_data.append(line) 50 open_p.append(float(split_line[1])) 51 close_p.append(float(split_line[4])) 52 if '-' in split_line[5]: 53 vol.append(0) 54 else: 55 vol.append(float(split_line[5])) 56 # Fix date and timing 57 split_date=split_line[0].split('-') 58 week_day = int(split_date[0]); 59 mon = month2num(split_date[1]); 60 yr = 2000 + int(split_date[2]) 61 date_temp = datetime.datetime(year=yr, month=mon, day=week_day) 62 trade_date.append(mdates.date2num(date_temp)) 63 # print(date[0].isoformat()) 64 # print(split_line[0]) 65 # print(stock_price_data[0:1]) 66 ax1.plot_date(trade_date, open_p, 'red') 67 plt.xlabel('Date') 68 plt.ylabel('Stock price, $') 69 plt.title('Stock price of '+ticker) 70 plt.show() 71 72 73 stock_data("NOK") 74